Machine Learning Engineer -
Competency Mapping
Introduction
Aspire Ability engaged with a large organization that was facing a talent crunch in data analytics and data science. Their stated objective was to hire dozens more “machine learning engineers” to add to their already sizable team. As one leader in the organization put it, they didn’t care about “soft skills.” They just wanted people who could write and implement algorithms.
They realized, however, that they weren’t as clear as they wanted to be about the specific competencies they needed in these jobs. Aspire Ability’s role in the process was to identify and articulate these competencies before they began trying to fill their newly created vacancies.
Like many companies in the same position, this organization faced two additional challenges: (1) there was substantial demand for people with these skills in their geographic region, making it difficult to be specific and particular about prospects’ skill sets, and (2) they were having difficulty keeping the people they did hire, particularly their most talented, senior people.
What We Did
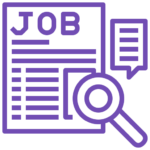
Our FIRST STEP was to learn more about the roles in question: How many people were in each role? How many are hired on a monthly, quarterly, and annual basis? What current recruiting practices were used with what rates of success?
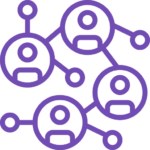
SECOND, we evaluated the roles in their organizational context, e.g., what was the team structure, were people in the same role colocated, were there levels in the roles, etc.
THIRD, we conducted interviews, reviewed current job descriptions, role KPIs and KSAs (if available) and factored in both organizational expectations and industry standards to craft a draft competency map.
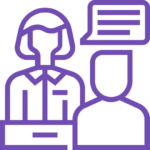
NEXT, we surveyed current incumbents in the roles, their peers, managers, and key stakeholders to evaluate, calibrate, and critique the draft competency map..
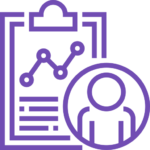
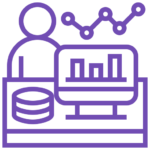
Our FIFTH STEP in the process was to thoroughly evaluate our survey data and share, probe, and validate our findings with key stakeholders in a series of focus groups.
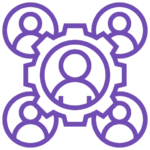
FINALLY, we synthesized all of the data and feedback to create “Version 1.0” of competency maps for the targeted roles. We presented our findings to the sponsors and HR stakeholders and made recommendations.
Results
Based on our analyses, we drew three key conclusions about the talent context of this organization:
- The lack of clarity around the targeted data analytics roles made the external hiring and internal promotion process opaque, mysterious, inconsistent, and frustrating;
- While our project focused on two key data analytics roles, some of the frustrations and even turnover on the team was the result of inadequate FTE allocation on the team to supporting and technical roles (leaving higher-paid, more analytical roles to perform many team’s product, project, and technical functions). It turns out teams have their own competency needs and “maps” (with individual competencies overlapping in a “cloud,” illustrated below);
- While managers were primarily focused on the technical skills of their data analytics team members, stakeholders in the business expressed a need for better soft skills, e.g., empathy and collaboration.
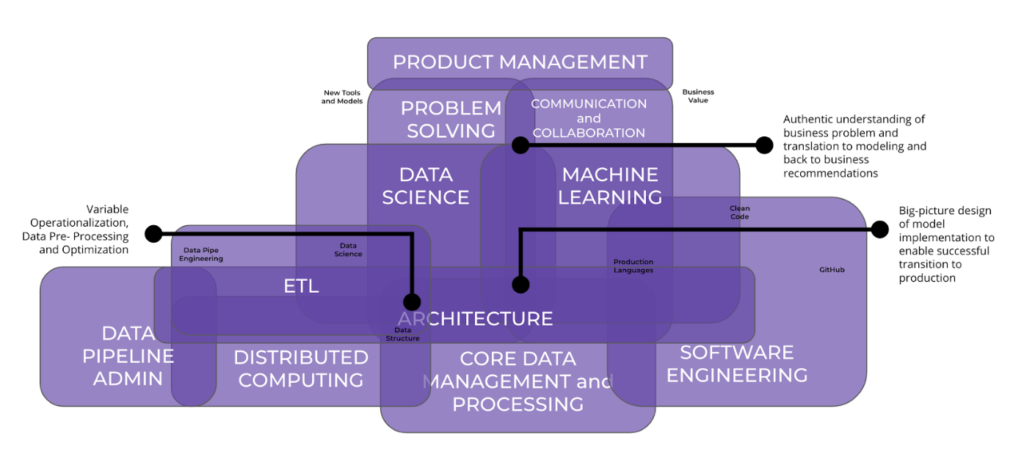
Accordingly, we recommended that the organization begin implementing more rigorous competency-based evaluations of internal and external job candidates as well as incumbents in their data analytics roles. We also recommended using these processes to build out a more diverse, competency-optimized team.